The energy in Vietnam’s cities is frenetic as millions of people navigate the busy streets with determination and purpose. Much of this traffic is driven by e-commerce, with food and parcel deliveries perched on the back of the country’s countless motorcycles or in cars and trucks. In the first quarter of 2024, online spending in Vietnam grew a staggering 79% over the previous year. Explosive growth like this is expected to continue, raising the industry’s value to $32 billion by 2025, with 70% of the country’s 100 million population making e-commerce transactions.
With massive numbers like this, in logistics, efficiency is king. The high customer expectations for rapid deliveries drive companies like Ahamove to innovate their way to seamless operations with cloud technology. Ahamove is Vietnam’s largest on-demand delivery company, handling more than 200,000 e-commerce, food, and warehouse deliveries daily, with 100,000 drivers and riders plying the streets nationwide. The logistics leader serves a network of more than 300,000 merchants, including regional e-commerce giants like Lazada and Shopee, as well as nationwide supermarket chains and small restaurants. The stakes are high for all involved, so maximizing efficiency is of utmost importance.
Innovating to make scale count
Online shoppers’ behavior is rarely predictable, and to cope with sudden spikes in daily delivery demand, Ahamove needed to efficiently scale up its operations to enhance customer and end-user satisfaction. Moving to MongoDB Atlas on Amazon Web Services (AWS) in 2019, Ahamove fundamentally changed its ability to meet the rising demand for deliveries and new services that please e-commerce providers, online shoppers, and diners.
The scalability of MongoDB is crucial for Ahamove, especially during peak times, like Christmas or Lunar New Year, when the volume of orders surges to more than 200,000 a day. “MongoDB's ability to scale ensures that the database can handle increased loads, including data requests, without compromising performance and leading to quicker order processing and improved user experience,” said Tien Ta, Strategic Planning Manager at Ahamove.
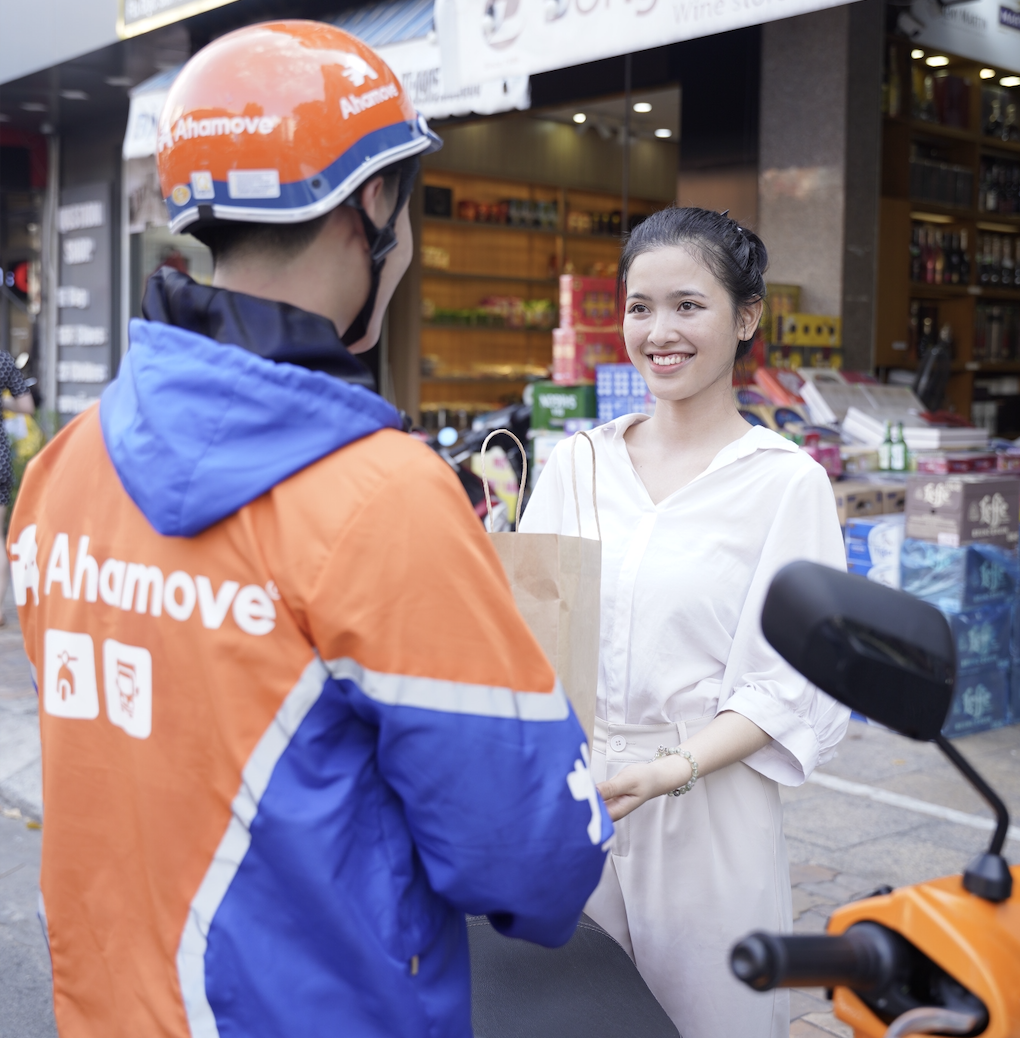
One of the powerful services that improves e-commerce across Vietnam is geospatial queries enabled by MongoDB. Using this geospatial data associated with specific locations on Earth's surface, Ahamove can easily locate drivers, map drivers to restaurants to accelerate deliveries, and track orders without relying on third-party services to provide information, which slows deliveries. Meanwhile, the versatility of MongoDB’s developer data platform empowers Ahamove to store its operational data, metadata, and vector embeddings on MongoDB Atlas and seamlessly use Atlas Vector Search to index, retrieve, and build performant generative artificial intelligence (AI) applications.
AI evolution
Powered by MongoDB Atlas, Ahamove is transforming Vietnam’s e-commerce industry with innovations like instant order matching, real-time GPS vehicle tracking, generative AI chatbots, and services like driver rating and variable delivery times, all available 24 hours a day, seven days a week.
In addition to traffic, Vietnam is also famous for its excellent street food. Recognizing the importance of the country’s rapidly growing food and beverage (F&B) industry, which is projected to be worth more than US$27.3 billion in 2024, Ahamove decided to help Vietnam’s small food vendors benefit from the e-commerce boom gripping the country. Using the latest models, including ChatGPT-4o-mini and Llama 3.1, Ahamove’s fully automated generative AI chatbot on MongoDB integrates with restaurants’ Facebook pages. This makes it easier for hungry consumers to handle the entire order process with the restaurant in natural language, from seeking recommendations to placing orders, making payments, and tracking deliveries to their doorsteps.
“Vietnam’s e-commerce industry is growing rapidly as more people turn to their mobile devices to purchase goods and services,” added Ta. “With MongoDB, we meet this customer need for new purchase experiences with innovative services like generative AI chatbots and faster delivery times.”
Anticipated to achieve 10% of food deliveries at Da Nang market and take the solution nationwide in the first half of 2025, AhaFood.AI - Ahamove’s latest initiative, also provides personalized dish recommendations based on consumer demographics, budgets, or historical preferences, helping people find and order their favorite food faster. Moreover, merchants receive timely notifications of incoming orders via the AhaMerchant web portal, allowing them to start preparing dishes earlier. AhaFood.AI also collects and securely stores users’ delivery addresses and phone numbers, ensuring better driver assignment and fulfilling food orders in less than 15 minutes.
“Adopting MongoDB Atlas was one of the best decisions we’ve ever made for Ahamove, allowing us to build an effective infrastructure that can scale with growing demand and deliver a better experience for our drivers and customers,” said Ngon Pham, CEO, Ahamove. “Generative AI will significantly disrupt the e-commerce and food industry, and with MongoDB Vector Search we can rapidly build new solutions using the latest database and AI technology.”
The vibrant atmosphere of Vietnam's bustling cities is part of the country's charm. Rather than seeking to bring calm to this energy, Vietnam thrives on it. Focusing on improving efficiency and supporting street food vendors in lively urban areas with cloud technology will benefit all.
Learn how to build AI applications with MongoDB Atlas.
Head over to our quick-start guide to get started with Atlas Vector Search today.