Aftersales service is a crucial revenue stream for the automotive industry, with leading manufacturers executing repairs through their dealer networks. One global automotive giant recently embarked on an ambitious project to revolutionize their diagnostic process. Their project—which aimed to increase efficiency, customer satisfaction, and revenue throughput—involved the development of an AI-powered solution that could quickly analyze engine sounds and compare them to a database of known problems, significantly reducing diagnostic times for complex engine issues.
Traditional diagnostic methods can be time-consuming, expensive, and imprecise, especially for complex engine issues. MongoDB’s client in automotive manufacturing envisioned an AI-powered solution that could quickly analyze engine sounds and compare them to a database of known problems, significantly reducing diagnostic times.
Initial setbacks, then a fresh perspective
Despite the client team's best efforts, the project faced significant challenges and setbacks during the nine-month prototype phase. Though the team struggled to produce reliable results, they were determined to make the project a success.
At this point, MongoDB introduced its client to Pureinsights, a specialized gen AI implementation and MongoDB AI Application Program partner, to rethink the solution and to salvage the project. As new members of the project team, and as PureInsights’s CTO and Lead Architect, respectively, we brought a fresh perspective to the challenge.
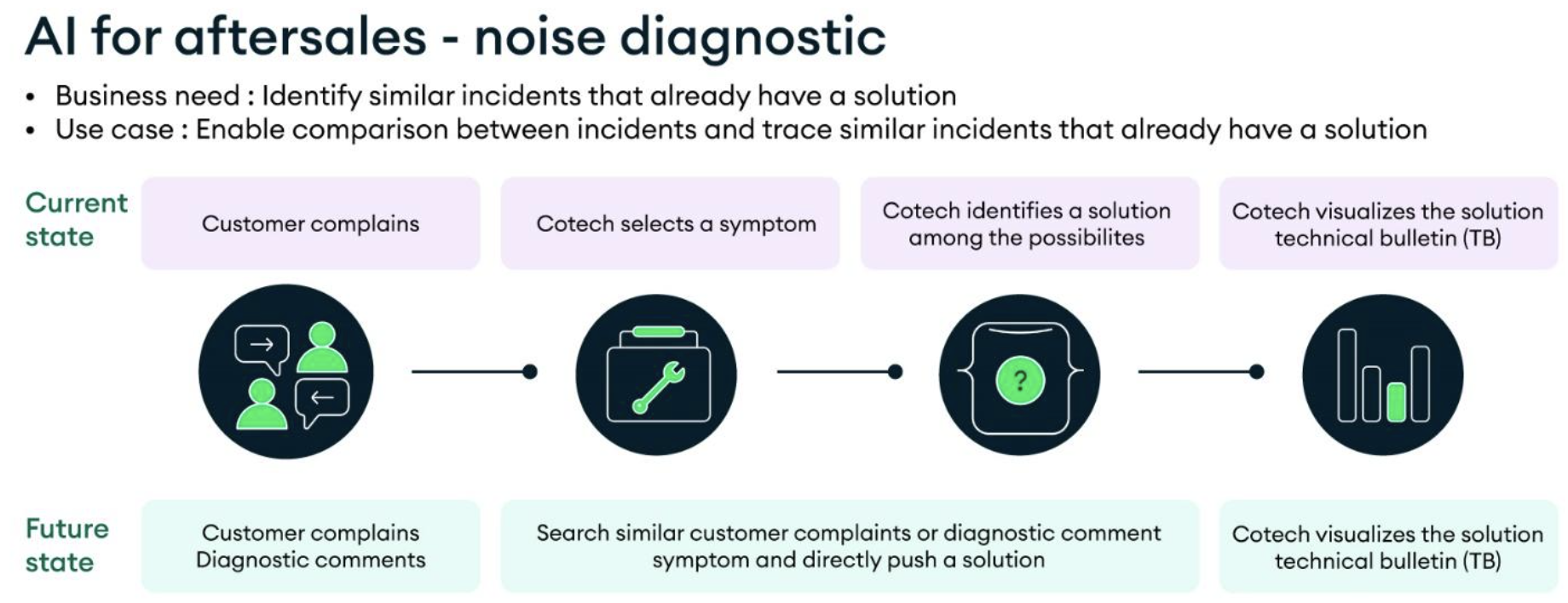
A pragmatic approach: Text before sound
Upon review, we discovered that the project had initially started with a text-based approach before being persuaded to switch to sound analysis. The PureInsights team recommended reverting to text analysis as a foundational step before tackling the more complex audio problem.
This strategy involved:
-
Collecting text descriptions of car problems from technicians and customers.
-
Comparing these descriptions against a vast database of known issues already stored in MongoDB.
-
Utilizing advanced natural language processing, semantic / vector search, and Retrieval Augmented Generation techniques to identify similar cases and potential solutions.
Our team tested six different models for cross-lingual semantic similarity, ultimately settling on Google's Gecko model for its superior performance across 11 languages.
Pushing boundaries: Integrating audio analysis
With the text-based foundation in place, we turned to audio analysis. Pureinsights developed an innovative approach to the project by combining our AI expertise with insights from advanced sound analysis research. We drew inspiration from groundbreaking models that had gained renown for their ability to identify cities solely from background noise in audio files.
This blend of AI knowledge and specialized audio analysis techniques resulted in a robust, scalable system capable of isolating and analyzing engine sounds from various recordings. We adapted these sophisticated audio analysis models, originally designed for urban sound identification, to the specific challenges of automotive diagnostics. These learnings and adaptations are also applicable to future use cases for AI-driven audio analysis across various industries.
This expertise was crucial in developing a sophisticated audio analysis model capable of:
-
Isolating engine and car noises from customer or technician recordings.
-
Converting these isolated sounds into vectors.
-
Using these vectors to search the manufacturer's existing database of known car problem sounds.
At the heart of this solution is MongoDB’s powerful database technology. The system leverages MongoDB’s vector and document stores to manage over 200,000 case files. Each "document" is more akin to a folder or case file containing:
-
Structured data about the vehicle and reported issue
-
Sound samples of the problem
-
Unstructured text describing the symptoms and context
This unified approach allows for seamless comparison of text and audio descriptions of customer engine problems using MongoDB's native vector search technology.
Encouraging progress and phased implementation
The solution's text component has already been rolled out to several dealers, and the audio similarity feature will be integrated in late 2024. This phased approach allows for real-world testing and refinement before a full-scale deployment across the entire repair network.
The client is taking a pragmatic, step-by-step approach to implementation. If the initial partial rollout with audio diagnostics proves successful, the plan is to expand the solution more broadly across the dealer network. This cautious (yet forward-thinking) strategy aligns with the automotive industry's move towards more data-driven maintenance practices.
As the solution continues to evolve, the team remains focused on enhancing its core capabilities in text and audio analysis for current diagnostic needs. The manufacturer is committed to evaluating the real-world impact of these innovations before considering potential future enhancements. This measured approach ensures that each phase of the rollout delivers tangible benefits in efficiency, accuracy, and customer satisfaction.
By prioritizing current diagnostic capabilities and adopting a phased implementation strategy, the automotive giant is paving the way for a new era of efficiency and customer service in their aftersales operations. The success of this initial rollout will inform future directions and potential expansions of the AI-powered diagnostic system.
A new era in automotive diagnostics
The automotive giant brought industry expertise and a clear vision for improving their aftersales service. MongoDB provided the robust, flexible data platform essential for managing and analyzing diverse, multi-modal data types at scale. We, at Pureinsights, served as the AI application specialist partner, contributing critical AI and machine learning expertise, and bringing fresh perspectives and innovative approaches. We believe our role was pivotal in rethinking the solution and salvaging the project at a crucial juncture.
This synergy of strengths allowed the entire project team to overcome initial setbacks and develop a groundbreaking solution that combines cutting-edge AI technologies with MongoDB's powerful data management capabilities. The result is a diagnostic tool leveraging text and audio analysis to significantly reduce diagnostic times, increase customer satisfaction, and boost revenue through the dealer network.
The project's success underscores several key lessons:
-
The value of persistence and flexibility in tackling complex challenges
-
The importance of choosing the right technology partners
-
The power of combining domain expertise with technological innovation
-
The benefits of a phased, iterative approach to implementation
As industries continue to evolve in the age of AI and big data, this collaborative model—bringing together industry leaders, technology providers, and specialized AI partners—sets a new standard for innovation. It demonstrates how companies can leverage partnerships to turn ambitious visions into reality, creating solutions that drive business value while enhancing customer experiences.
The future of automotive diagnostics—and AI-driven solutions across industries—looks brighter thanks to the combined efforts of forward-thinking enterprises, cutting-edge database technologies like MongoDB, and specialized AI partners like Pureinsights. As this solution continues to evolve and deploy across the global dealer network, it paves the way for a new era of efficiency, accuracy, and customer satisfaction in the automotive industry. This solution has the potential to not only revolutionize automotive diagnostics but also set a new standard for AI-driven solutions in other industries, demonstrating the power of collaboration and innovation.
To deliver more solutions like this—and to accelerate gen AI application development for organizations at every stage of their AI journey—Pureinsights has joined the MongoDB AI Application Program (MAAP). Check out the MAAP page to learn more about the program and how MAAP ecosystem members like Pureinsights can help your organization accelerate time-to-market, minimize risks, and maximize the value of your AI investments.